Mastering Image Labeling Tools for Classification: A Path to Data Annotation Excellence
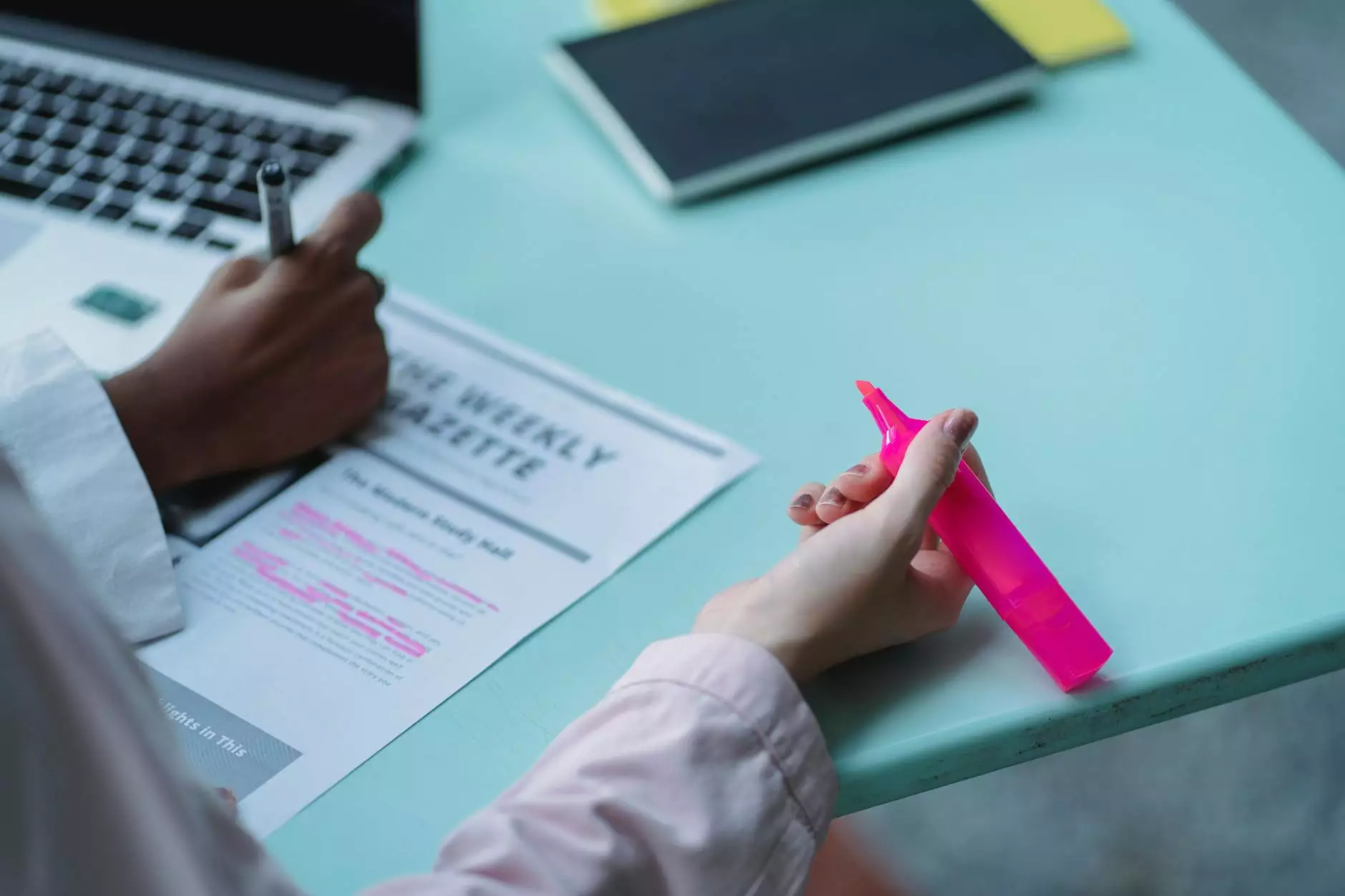
In today’s data-driven landscape, businesses are increasingly reliant on technology to harness the powers of artificial intelligence (AI) and machine learning (ML). A critical component of these advanced systems is the image labeling tool for classification. Such tools facilitate the process of accurately annotating images, which is essential for the training of robust machine learning models. In this article, we will delve into the importance of these tools, explore their functionalities, and understand how to leverage them effectively through platforms like Keylabs.ai.
Understanding Image Labeling
Image labeling refers to the process of assigning tags or labels to images to teach algorithms how to recognize patterns, objects, and scenes within those images. This process is vital for various applications including:
- Object detection: Identifying instances of objects within images.
- Image classification: Categorizing images into predefined classes.
- Semantic segmentation: Labeling each pixel of an image with a class.
- Instance segmentation: Differentiating between separate objects in an image even if they belong to the same class.
The Role of an Image Labeling Tool for Classification
Employing an image labeling tool for classification can greatly enhance the efficiency and accuracy of the labeling process. These tools come equipped with various features designed to streamline the workflow:
Key Features of Image Labeling Tools
- User-Friendly Interface: An intuitive design that simplifies the labeling process for users of all skill levels.
- Automated Labeling: Some advanced tools offer AI-assisted labeling capabilities, reducing time and effort.
- Collaboration Support: Facilitating teamwork with cloud-based solutions allowing multiple users to work simultaneously.
- Export Formats: Compatibility with various file formats (e.g., JSON, XML) to ensure easy integration with machine learning frameworks.
Why Image Labeling is Crucial for Machine Learning Projects
The significance of accurate image labeling cannot be overstated. Quality labeled data serves as the backbone of machine learning, directly impacting:
Model Performance
The performance of a machine learning model is directly proportional to the quality and quantity of its training data. Poorly labeled images can lead to:
- Inaccurate Predictions: Misclassification or failure to detect relevant features.
- Increased Training Time: More data is required to compensate for errors in labeling.
Business Decision Making
In industries like healthcare, automotive, and retail, accurate image classification can influence critical decisions such as:
- Improving Customer Experiences: Using image recognition for tailored services and recommendations.
- Enhancing Product Safety: Detecting anomalies in product images to prevent recalls.
How to Choose the Right Image Labeling Tool
With a plethora of image labeling tools available, selecting the right one for your needs can be overwhelming. Consider the following criteria:
Scalability
Your chosen tool should be able to scale with your project. Whether you need to label a few images or thousands, the tool must handle large datasets efficiently.
Customization Options
Every project has unique requirements. The ability to customize labels, workflows, and interfaces to fit specific needs can significantly enhance productivity.
Integration with Other Tools
Evaluate how well the labeling tool integrates with other systems such as data storage and machine learning platforms. This ensures a seamless workflow.
Leveraging Keylabs.ai for Your Data Annotation Needs
Keylabs.ai positions itself as a leading provider of data annotation solutions, offering a robust image labeling tool for classification that meets various industry needs. Here’s why you should consider Keylabs.ai:
Robust Annotation Features
Keylabs.ai provides an array of powerful annotation features, including:
- Multi-Class Annotation: Tagging images across multiple categories with precision.
- Quality Assurance Mechanisms: Built-in tools for monitoring label accuracy and consistency.
AI-Assisted Labeling Functionality
With AI-assisted features, Keylabs.ai allows for quicker labeling by suggesting labels based on existing data, thus reducing the amount of manual work required.
Training and Support
Customers at Keylabs.ai benefit from extensive training materials and responsive support teams to ensure users can maximize their tools and achieve their objectives.
The Future of Image Labeling in AI Development
The future of image labeling is set to evolve rapidly with advancements in AI technologies. Here are some trends to watch:
Increased Automation
As machine learning models become more sophisticated, we can expect to see greater levels of automation in image labeling processes. Automated tools will continue to reduce human effort while improving accuracy.
Collaborative Efforts in Data Annotation
Collaboration between AI and human annotators will become increasingly important, creating a hybrid approach that combines the speed of automation with the precision of human insight.
Conclusion
The importance of a high-quality image labeling tool for classification is undeniable in our technology-driven world. Investing in the right tool not only enhances the quality of data but also contributes positively to the overall efficiency and effectiveness of AI-driven projects. By leveraging innovative platforms like Keylabs.ai, businesses can revolutionize their data annotation processes and drive success through better machine learning models.
Embracing the future of data annotation with comprehensive tools and solutions is imperative for organizations aiming to thrive in a competitive landscape. Take the next step towards excellence in data labeling and classification with Keylabs.ai today!